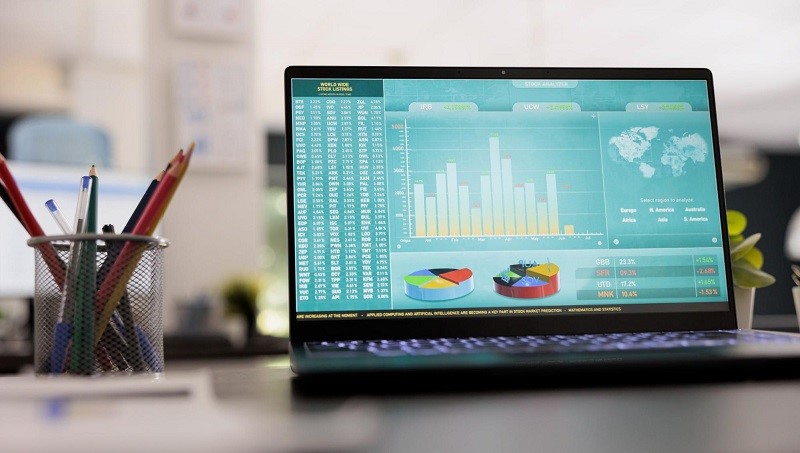
Algorithmic trading, or algo trading, leverages computer programs to execute trades at high speeds and frequencies. The success of an algorithm is often predicated on a backtesting platform, which involves running the algorithm on historical market data to assess its performance. However, the realism of these market conditions in backtesting is crucial for several reasons. Ensuring realistic market conditions can significantly influence the accuracy and reliability of the algo backtest results, thereby affecting the eventual success of the trading strategy in real-world scenarios.
Understanding Backtesting
Algo backtesting is a method used to evaluate a trading strategy by applying it to historical data. The primary goal is to see how the strategy would have performed in the past, providing insights into its potential effectiveness in future trading. The process involves:
- Data Collection: Gathering historical price data and other relevant market information.
- Strategy Implementation: Coding the trading algorithm based on predefined rules.
- Simulation: Running the algorithm on the historical data to simulate trades.
- Performance Evaluation: Analysing metrics such as profit and loss, risk-adjusted returns, drawdowns, and more.
While backtesting on a backtesting platform like uTrade Algosl, the accuracy of its results heavily depends on the realism of the simulated market conditions.
Why Realistic Market Conditions Matter
Market Liquidity and Slippage
- Liquidity refers to the ability to buy or sell an asset without causing a significant impact on its price. In real markets, liquidity varies, and low liquidity can lead to slippage—where the execution price differs from the intended price.
- In backtesting algorithmic trading, if the algos assumes perfect liquidity (i.e., every trade is executed at the desired price), the results may be overly optimistic. Realistic backtesting should incorporate varying liquidity levels and model potential slippage to reflect true market conditions.
Bid-Ask Spread
- The bid-ask spread is the difference between the highest price a buyer is willing to pay (bid) and the lowest price a seller is willing to accept (ask). This spread can affect trading costs and chances of overall profitability.
- Ignoring the bid-ask spread in backtesting can lead to an overestimation of the possible profits. Realistic backtesting includes these spreads, ensuring that the algorithm accounts for the actual costs of entering and exiting trades.
Market Impact
- Large trades can influence market prices, known as market impact. This is especially relevant for algorithms trading significant volumes relative to the market’s typical trading volume.
- A realistic backtest, on algo trading backtesting platforms, like uTrade Algos, should consider how large orders might affect prices and potentially lead to worse execution prices for subsequent trades.
Order Types and Execution
- Different order types (market, limit, stop-loss) and execution venues (exchanges, dark pools) have distinct behaviours and fill probabilities.
- In backtesting, simulating various order types and their respective execution probabilities adds realism. It helps in understanding how the algorithm would perform with different order execution scenarios.
Latencies and Delays
- Real-world trading involves various latencies—network delays, order processing times, and exchange latencies. These delays can affect the timing and execution of trades.
- Incorporating latencies into algo backtesting provides a more accurate picture of the algorithm’s performance, especially for high-frequency trading strategies where milliseconds matter.
Regulatory and Market Environment Changes
- Historical data may not account for changes in regulations, market structure, or economic events that could impact trading strategies.
- Realistic backtesting should consider how past regulatory and structural changes affected market conditions and model potential future changes.
Benefits of Realistic Backtesting
- Improved Accuracy: Realistic backtesting leads to more accurate performance metrics. By incorporating real-world factors, traders can better gauge the potential success of their strategies.
- Risk Management: It allows for better risk assessment and management. Understanding how an algorithm reacts to various market conditions helps in identifying potential risks and mitigating them effectively.
- Robust Strategy Development: Strategies on algo trading platforms, like uTrade Algos, tested under realistic conditions are more robust and adaptable. They are better equipped to handle the unpredictability of live markets.
- Enhanced Credibility: Results from realistic backtests are more credible to investors and stakeholders. It builds confidence in the strategy’s viability and performance potential.
- Regulatory Compliance: Realistic backtesting helps ensure that trading strategies comply with regulatory requirements, as many regulations necessitate that trading models are tested under realistic market conditions.
Key Considerations for Effective Backtesting in Algo Trading
- Data Quality and Accuracy: Use reliable, clean, and complete historical data in backtesting algorithmic trading to ensure that the results are accurate and trustworthy.
- Transaction Costs: Include realistic estimates of commissions, fees, and slippage to reflect true trading costs.
- Look-Ahead Bias: Ensure the strategy only uses data available at the time of each trade to avoid unrealistic performance results.
- Market Impact: Simulate the effect of large orders on market prices to understand how trade sizes influence execution.
- Order Execution Models: Use different order types and their fill probabilities to mimic real-world trading scenarios.
- Latency and Execution Delays: Factor in network and processing delays to accurately model the timing of trade executions.
In the world of algorithmic trading on algo trading backtesting platforms, like uTrade Algos, the importance of realistic market conditions in backtesting cannot be overstated. Unrealistic assumptions on backtesting platforms, in India and elsewhere across the world, can lead to overestimating the potential success of a trading strategy, resulting in significant financial losses when deployed in live markets. By incorporating factors such as market liquidity, bid-ask spreads, market impact, order execution types, latencies, and regulatory changes, traders can achieve a more accurate and reliable assessment of their strategies. Ultimately, this leads to better risk management, more robust strategies, and greater confidence in the trading algorithms developed.